ODSC's goal is to bring together the global data science community in an effort to encourage the exchange of innovative ideas and the growth of open source software.
Background
Since 2014, ODSC grew from one small Boston event to a Global Brand holding events for up to 5000 data inclined professionals at a time. During my time at ODSC, we launched a webinar service, a podcast, a learning platform and a career platform on top of a growing in-person event offering.
Experience Design Research (XD)
The sequence of headers shown hereafter map to blueprint CX. Click them to jump to that section and the back stage processes that may accompany them.
SALES, RESEARCH, PLAN, FIND YOUR WAY, ADMIRE, LEARN, MAKE FRIENDS
At the onset, a hypothesis journey helped reveal recruitment profiles and set personas in motion.
Brand Identity Transition
Emails and media campaigns
SALES
Before the on-site experience, to be attendees interact with ads.
Design decisions were influenced by a set of Design Principles—Inclusive, Clean, Accessible, Bright—that embodied ODSC’s smart, open and forward looking ideology, as well as by data pooled and analyzed by myself and the data science team.
Previous to the design principles, ODSC did not have brand guidelines. However, we did use a variety of blue shades throughout the brand, so I made sense of those design choices by first introducing a gradient background.
This 2018 video advertisement predated the Design Principles.
This 2019 advertisement concept developed in coordination with Warbird Creative. City sound data gradually becomes structured into an inspirational pattern.
Dark to Light to Contrast
Over time the overall web presence was lead to transition through making research from dark, to light, then to colorful contrast in accordance with these principles.
A masculine purple was chosen for our business-focused persona and a bright green often associated with innovation and sustainability for those focused on applied data science.
Web Experience
RESEARCH
After becoming aware of ODSC, users buy conference passes on ODSC.com and consume educational instructionals, created by the Data Science Team, on opendatascience.com.
Product Design and Design Research functions were built atop and channeled into instructional production cycles. The Data Scientists involved in this intrepreneurship lab, were led to create technologies, do analysis we could use and write about it.
Codeless solutions for odsc.com were constructed in high fidelity on a staging site with a drag and drop web-builder application. A record of odsc.com landing page as I designed it can be found at the link.
Collateral Examples
This is a walkthrough of opendatascience.com under development. This was an incremental rebuild of the whole site from the running state in March 2016. Designs were framed up on paper and through adobe products, then documented for a remote development team through annotated google development tool screenshots.
PLAN
To help manage their on-site experience to be attendees interact with planning artifacts — FAQs, and an app where they can chat with peers and select the workshops and talks they would like to attend.
On-Site Experience
internal experience documents
FIND YOUR WAY
Exhibitor Booth Pool
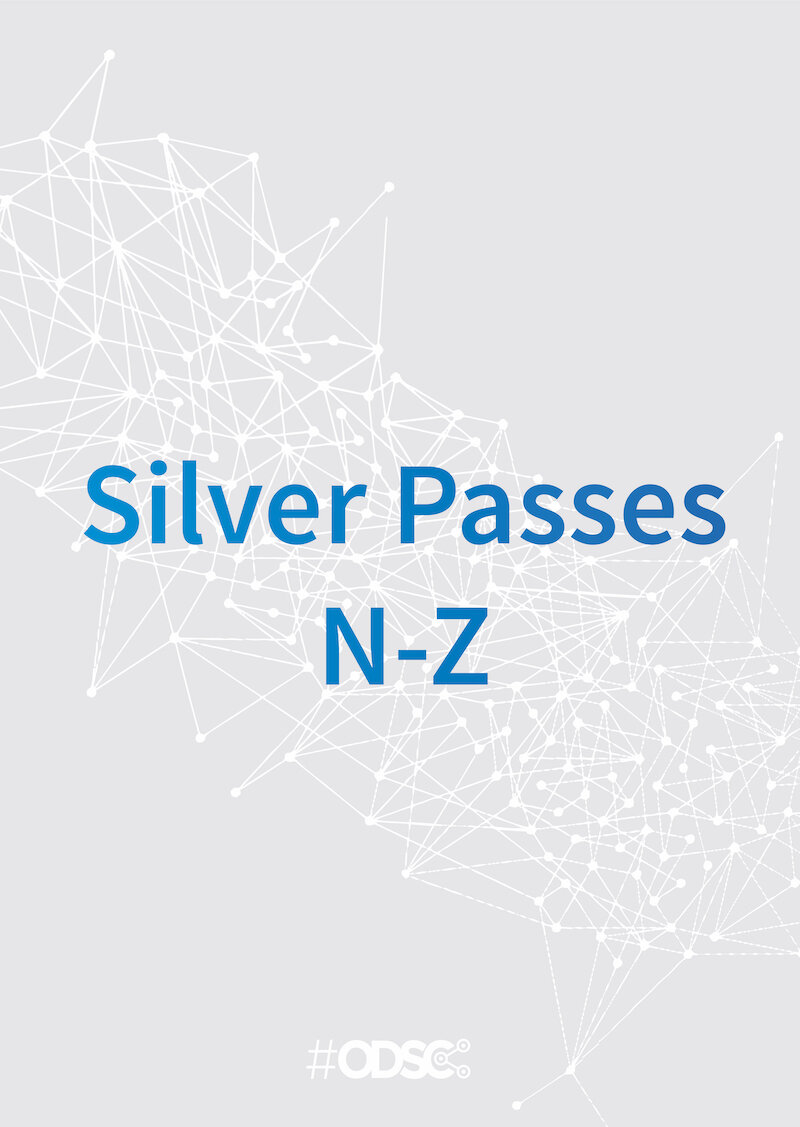
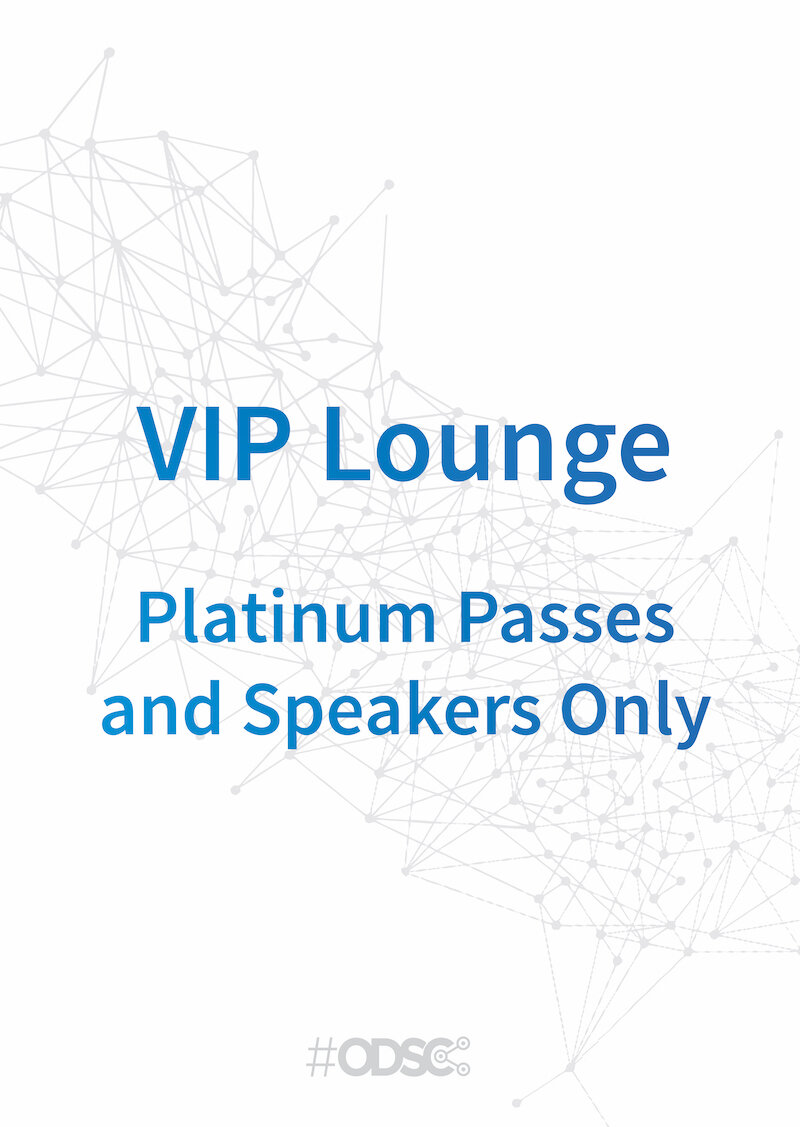
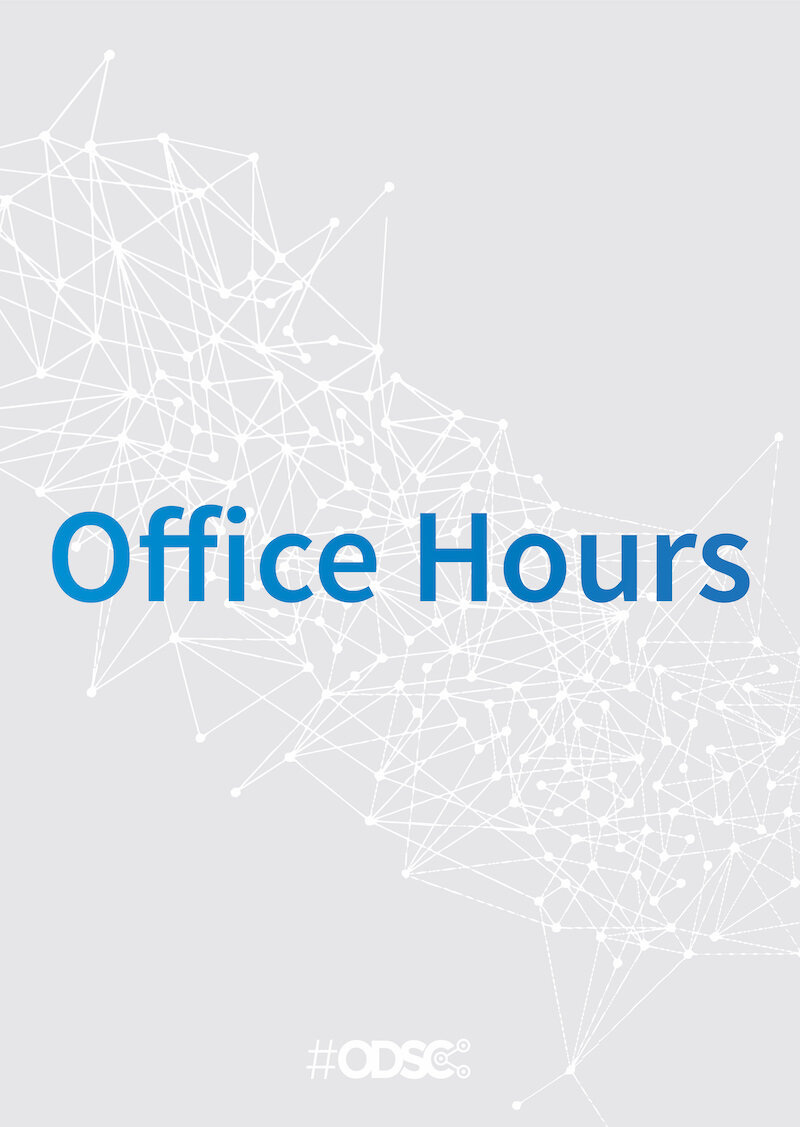
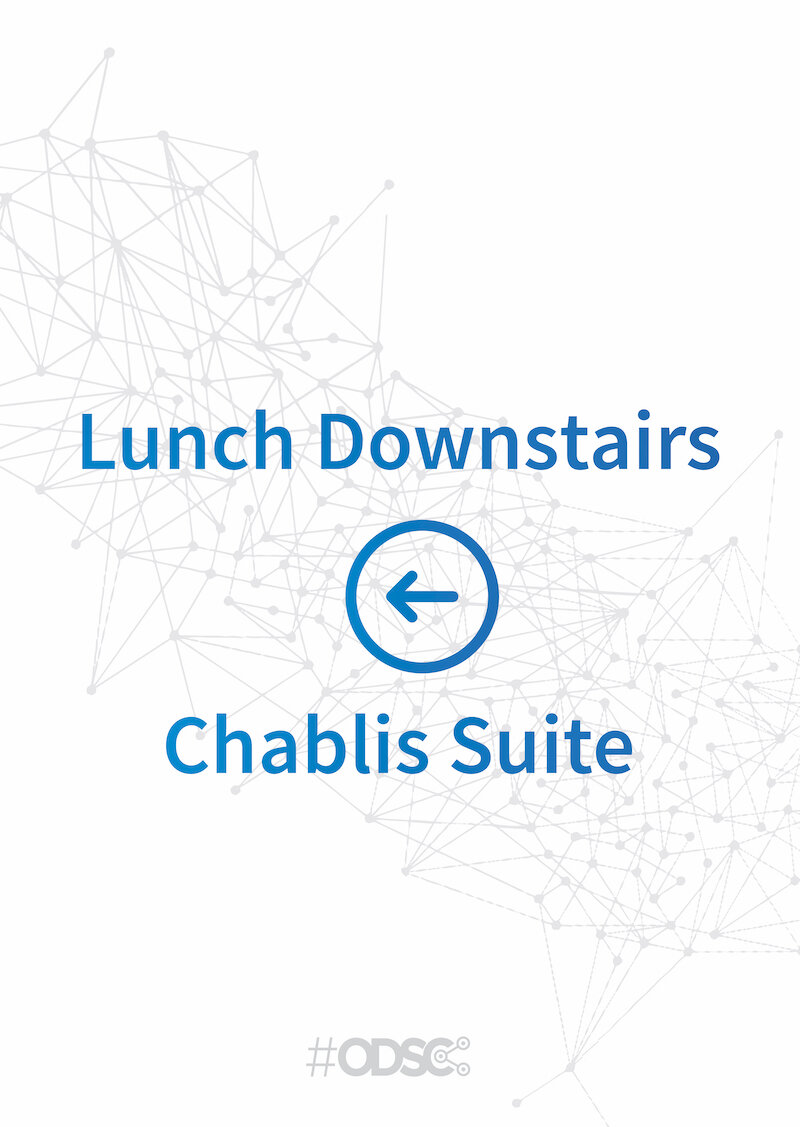
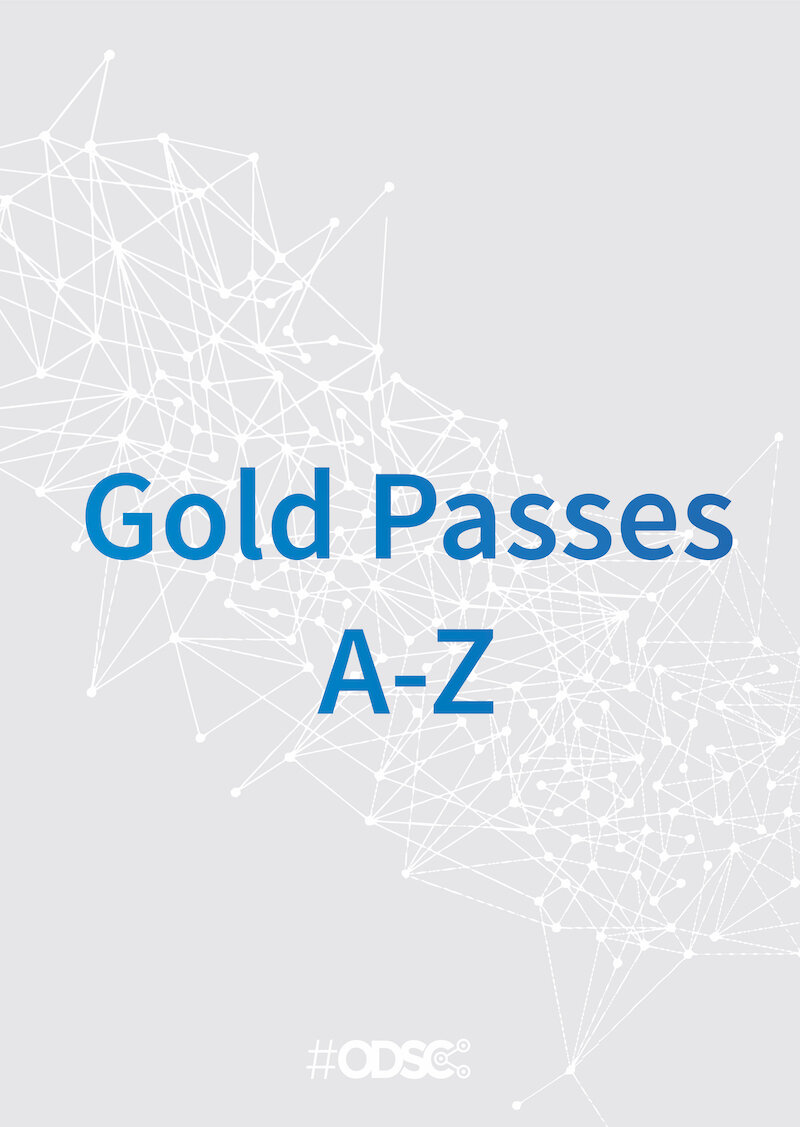
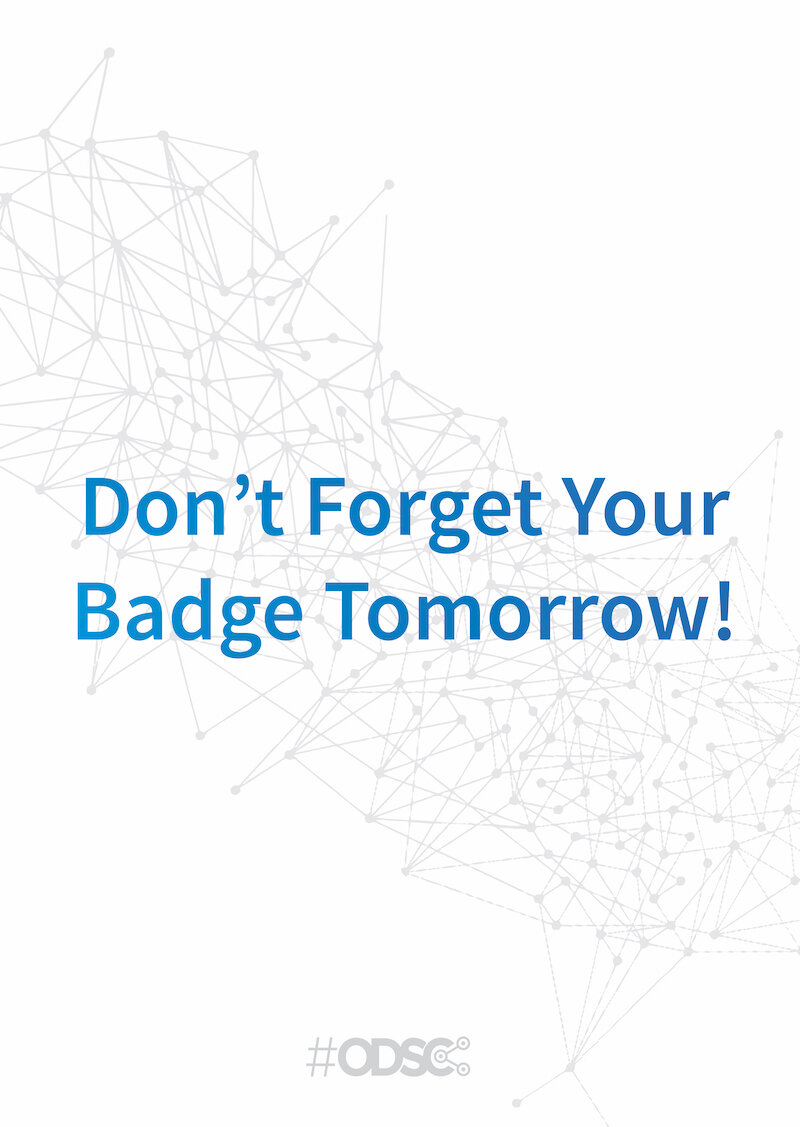
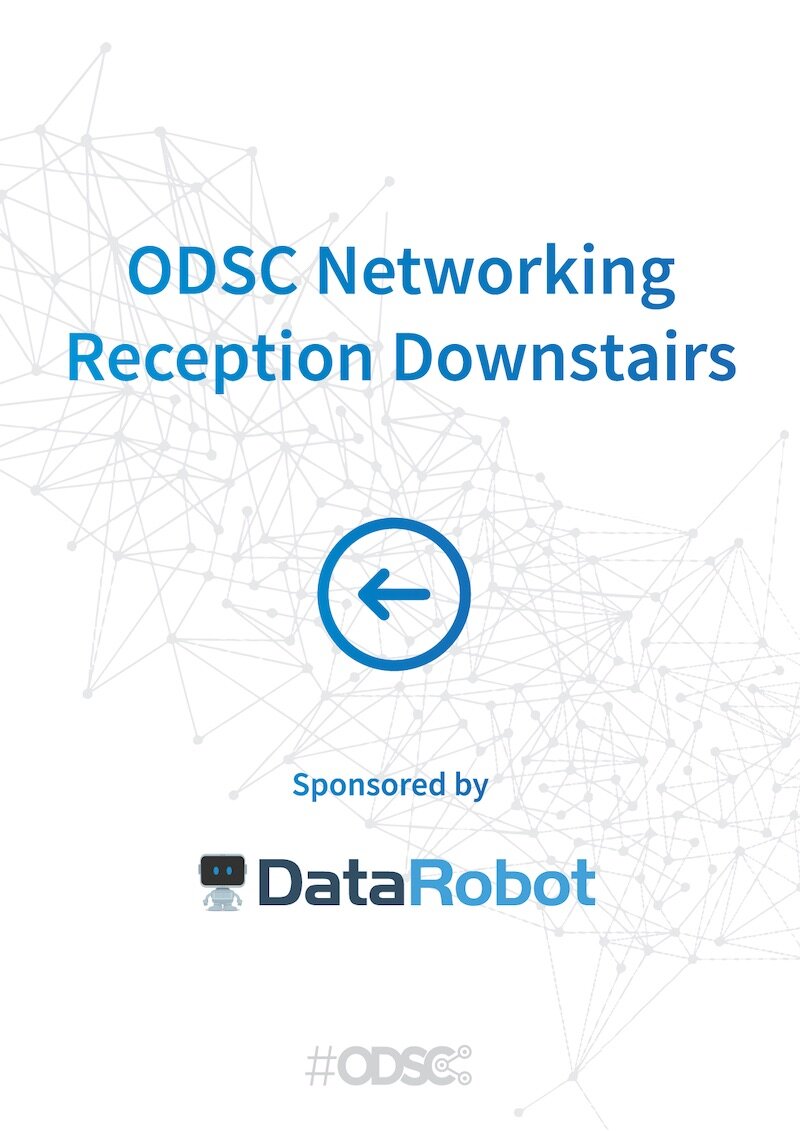
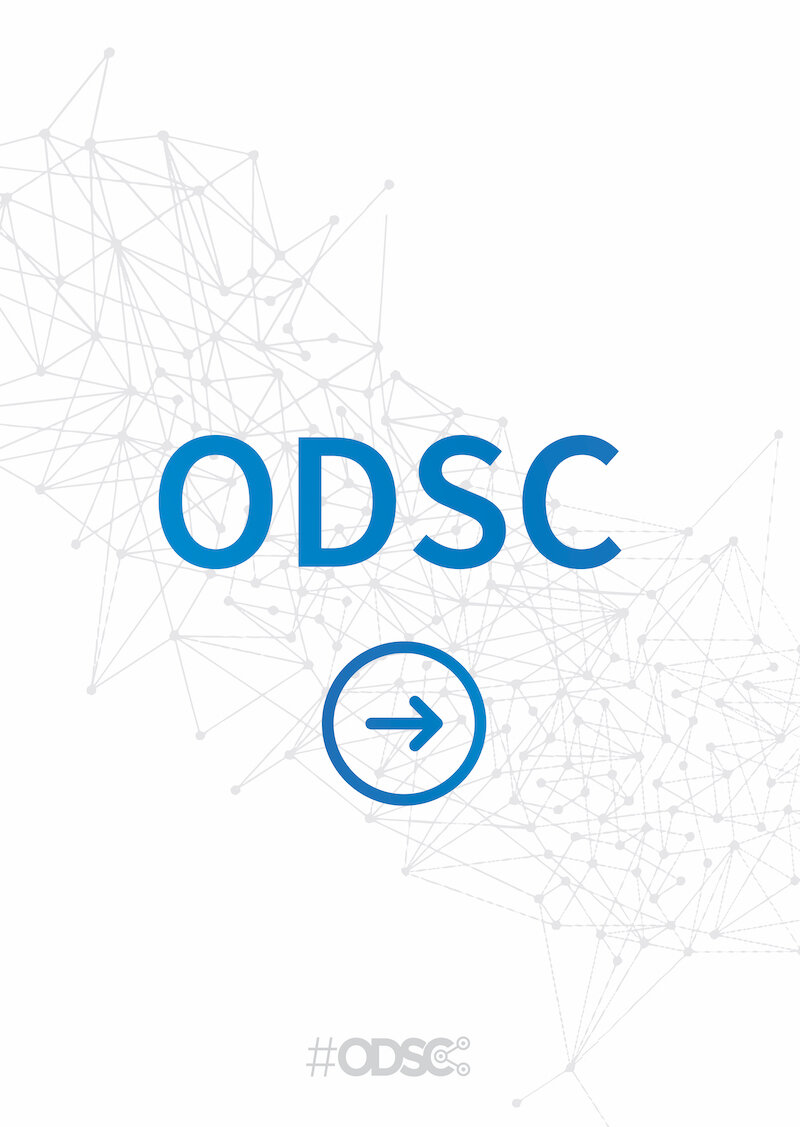
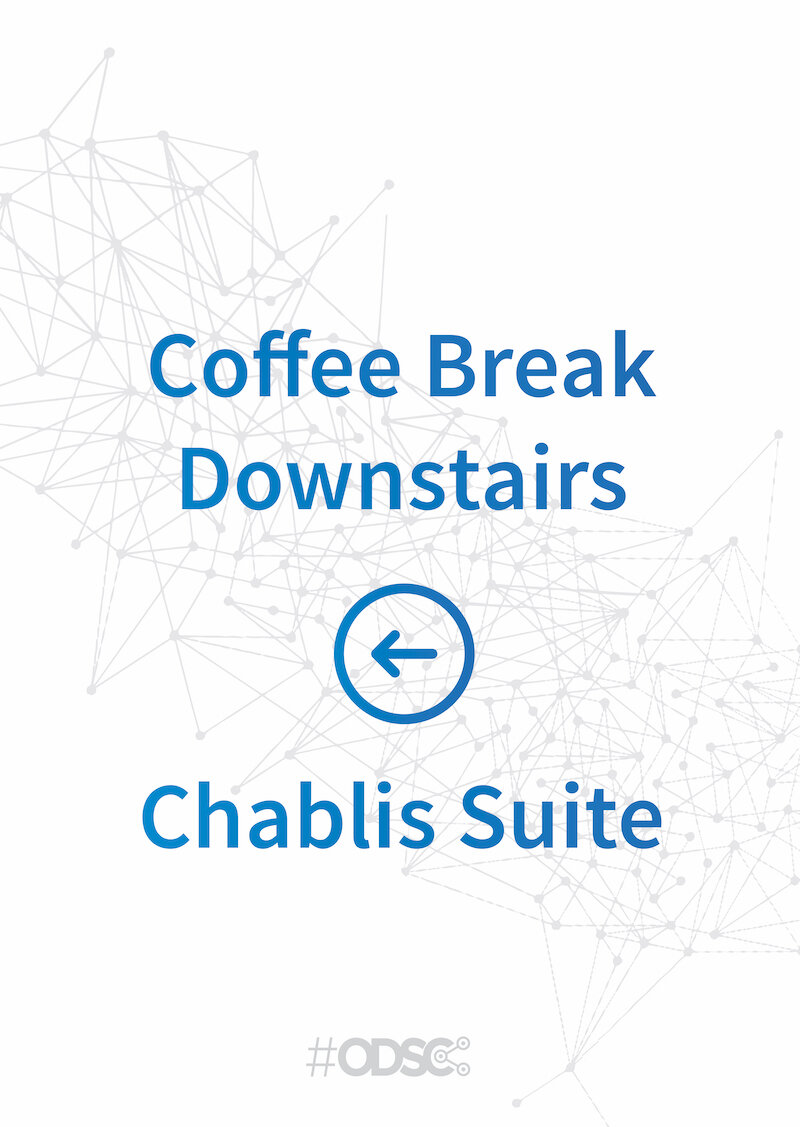
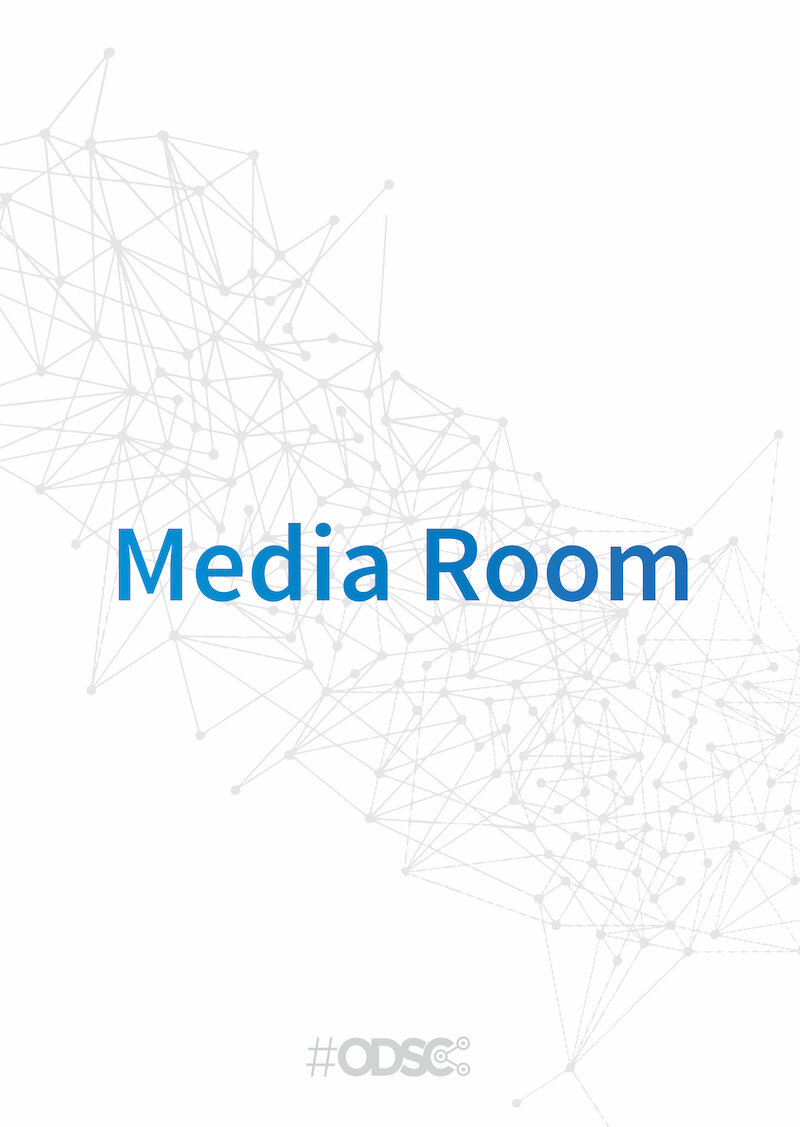
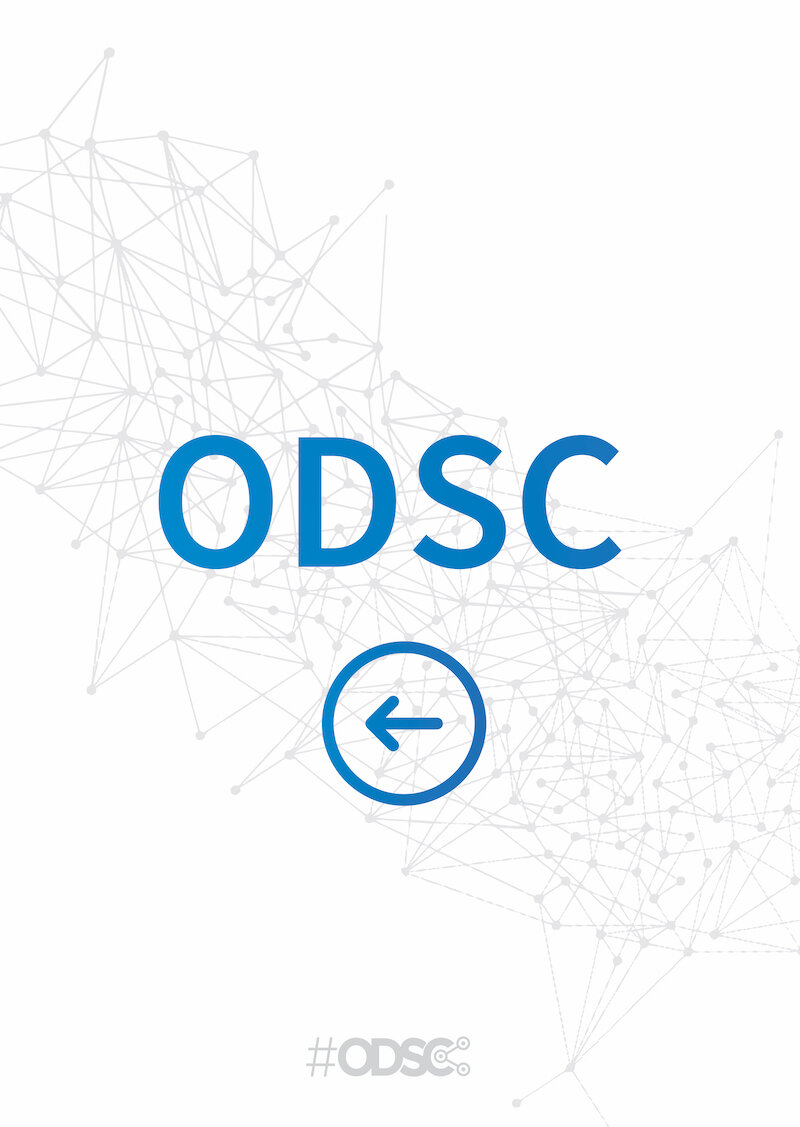
Pull up banners of the expanded ODSC service offering.
TV Schedule Sample
ADMIRE
The aspiration to become a speaker was realized in observation of speakers and user groups.
keynote S-banner backdrop and graphic
Podium Detail - laser cut logo fixed to wood
Podium Detail - networking graphic on white board cut to shape
LEARN
Between the London and California events we recruited 3 users for an in-depth interview and card sorting exercise, then, we supplemented those data with additional interviews — 5 dual-purpose SME interviews that were edited down for a podcast series and 20 short 5-10 minute interviews with 20 more attendees. Data scientist interviewers were paired with SMEs for interviews as part of a career development and design research skill building plan. In addition, we contracted photographer-designers to create photo-essay persona-journey artifacts of the conference experience. Synthesis was channeled through the design of archetypes, web pages, email and media campaigns, content and sales literature in post.
Archetypal Experience Dipdic #1
The number of stickers, what kind and what year they were given became a way to parse out frequent attenders of other conferences for intercept interviews.
MAKE FRIENDS
Archetypal Experience Dipdic #2
post talk networking drink voucher
Appendix
Sales Literature Example
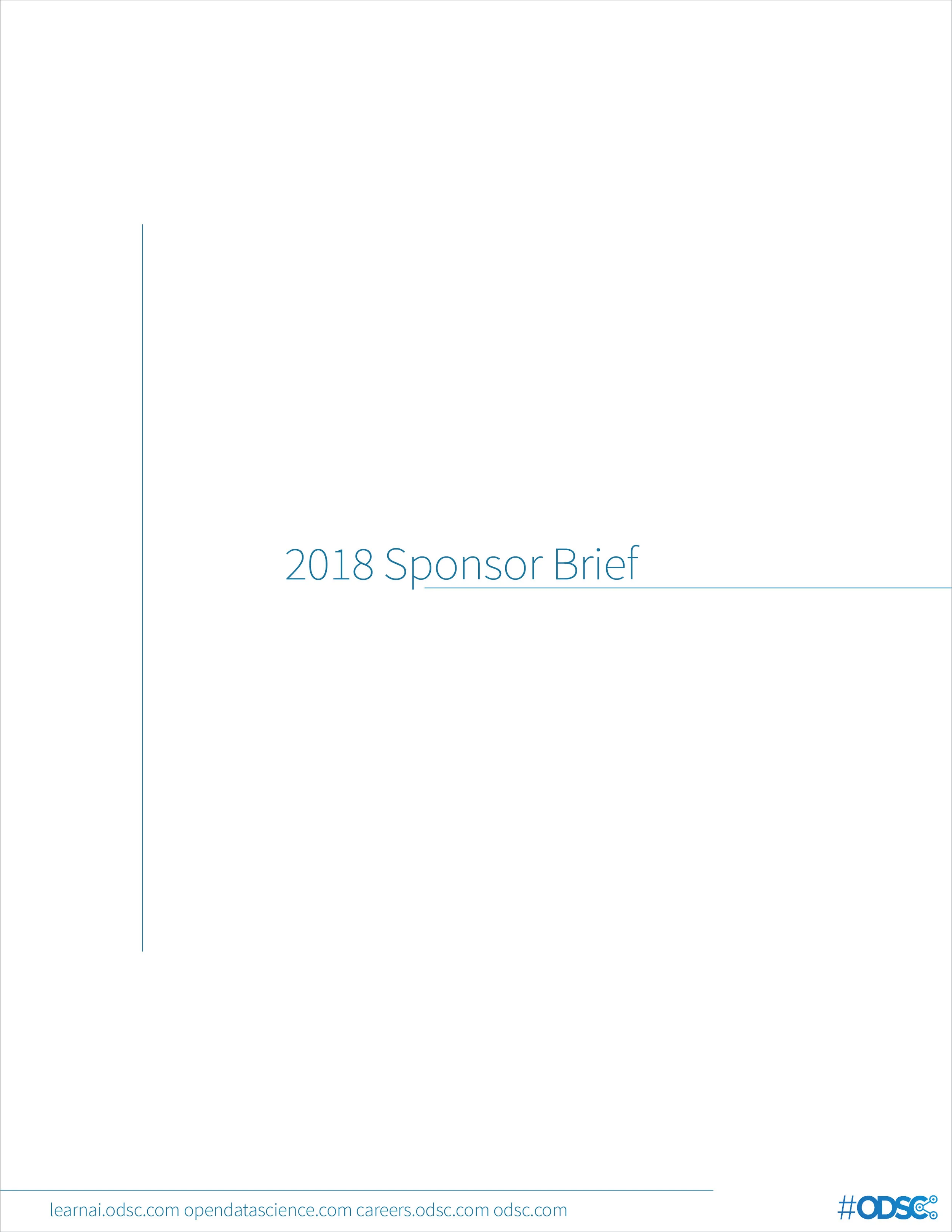
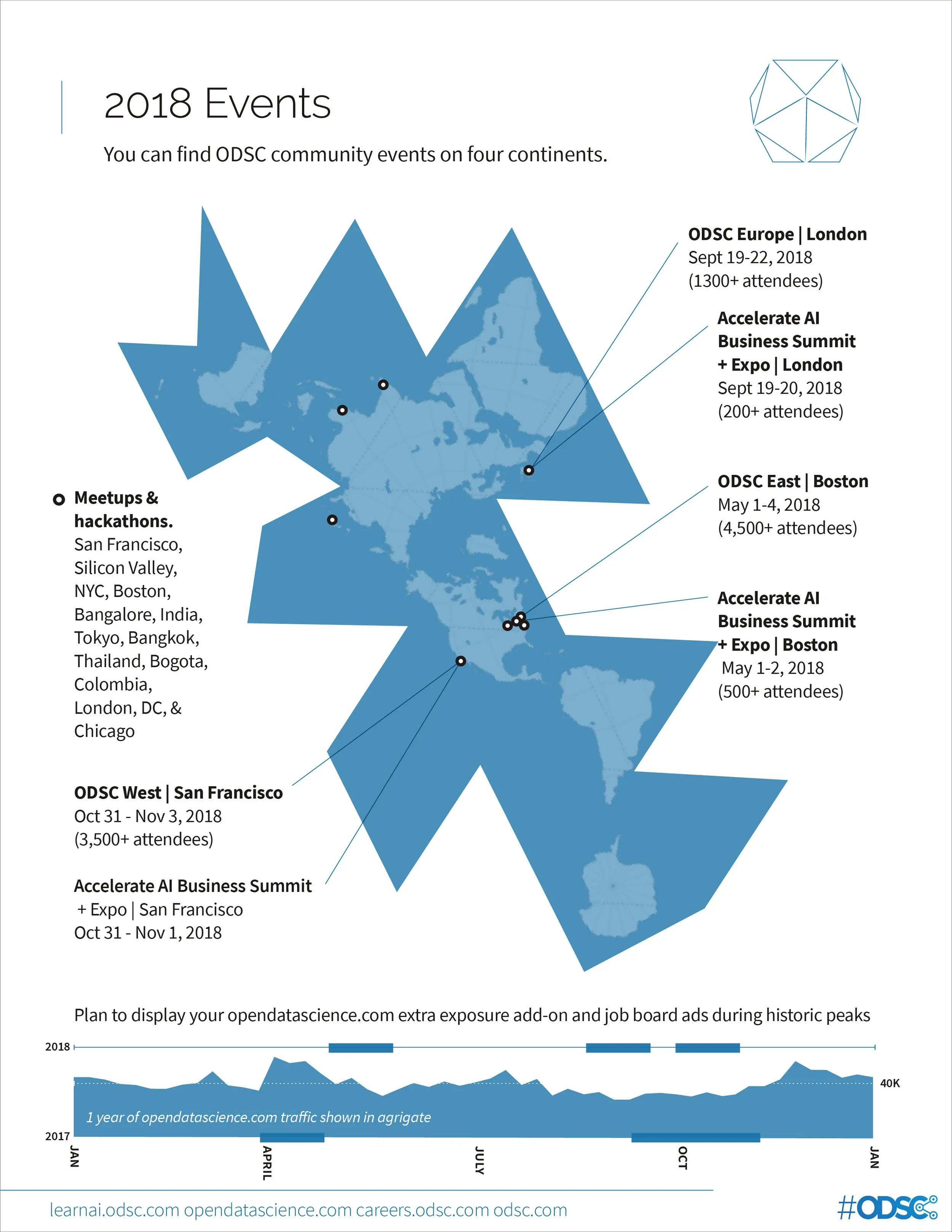
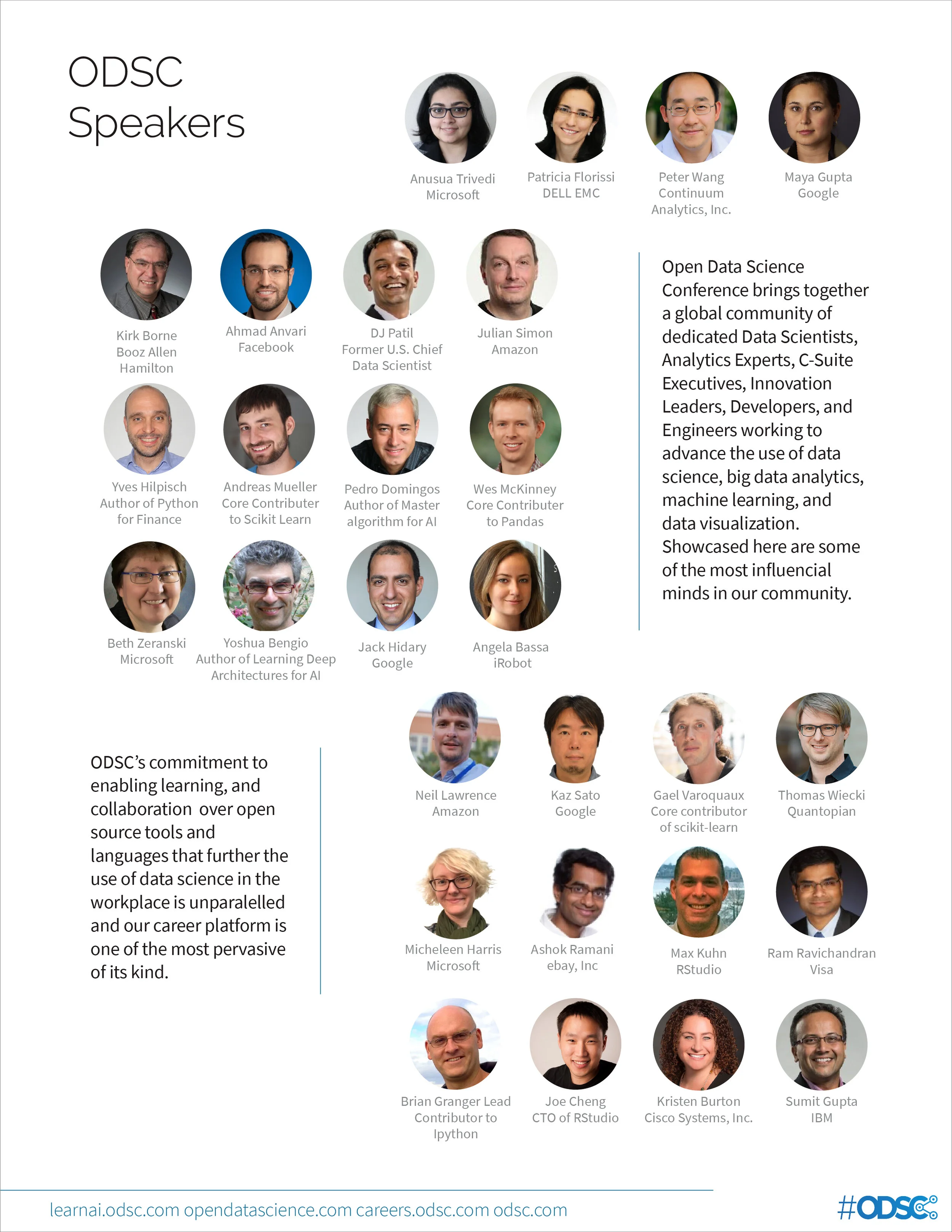

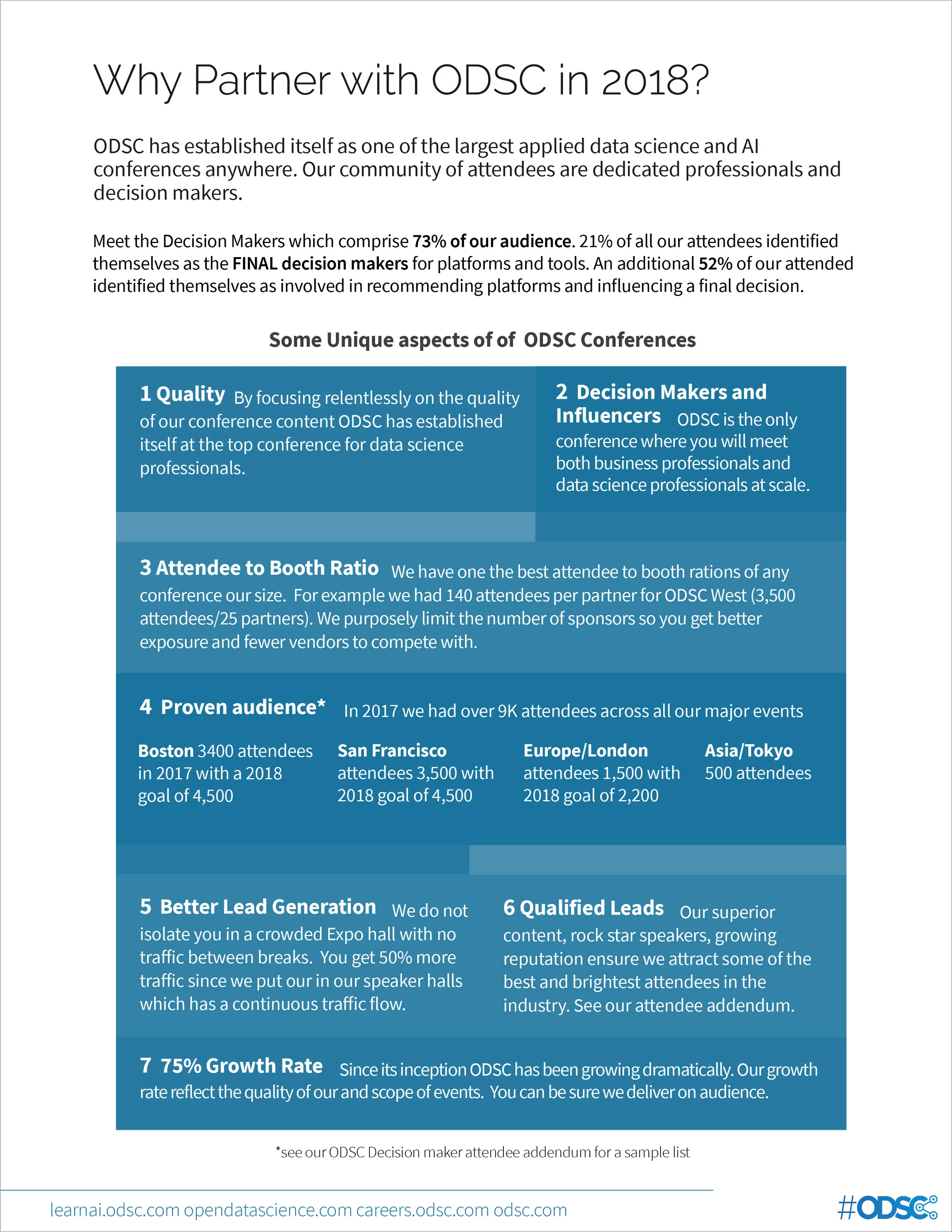
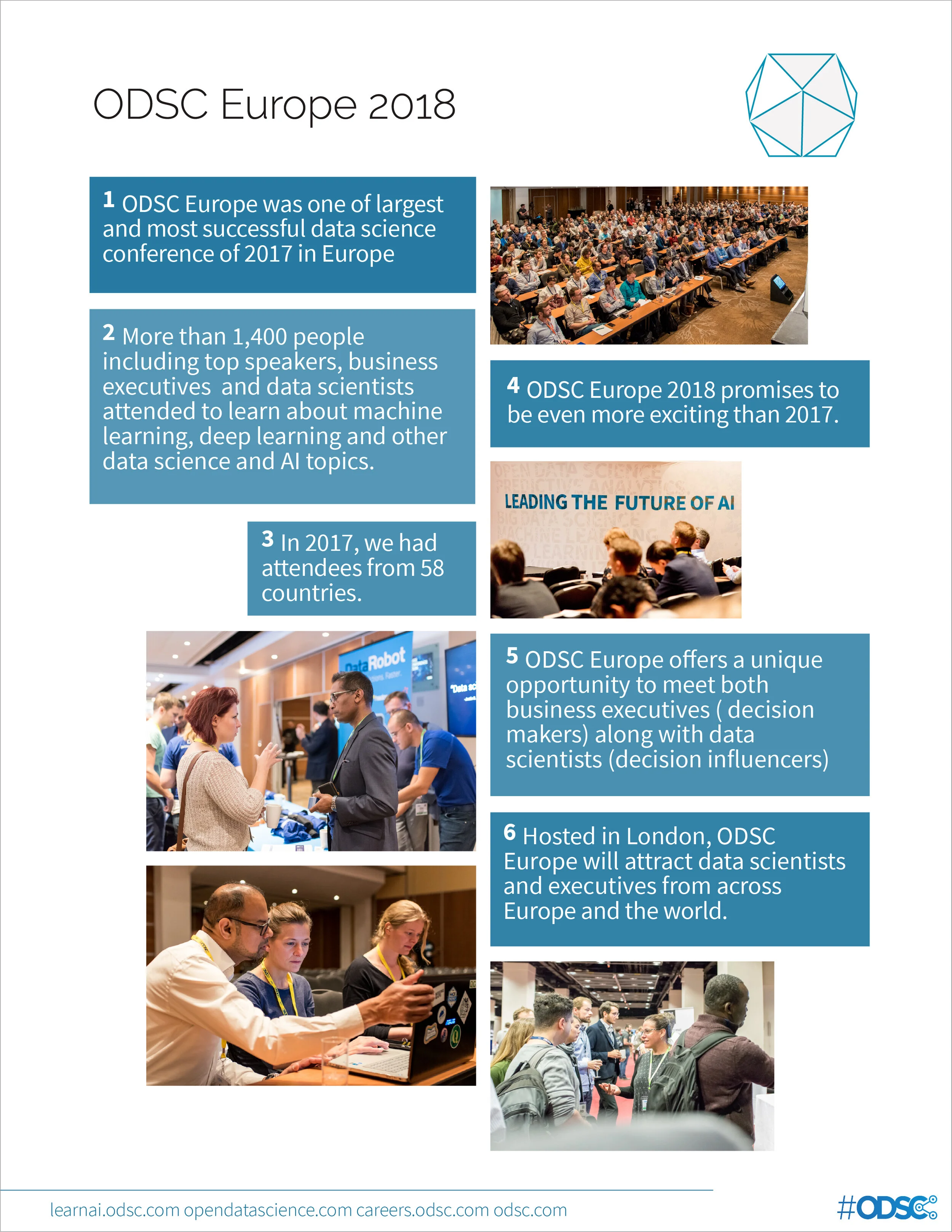

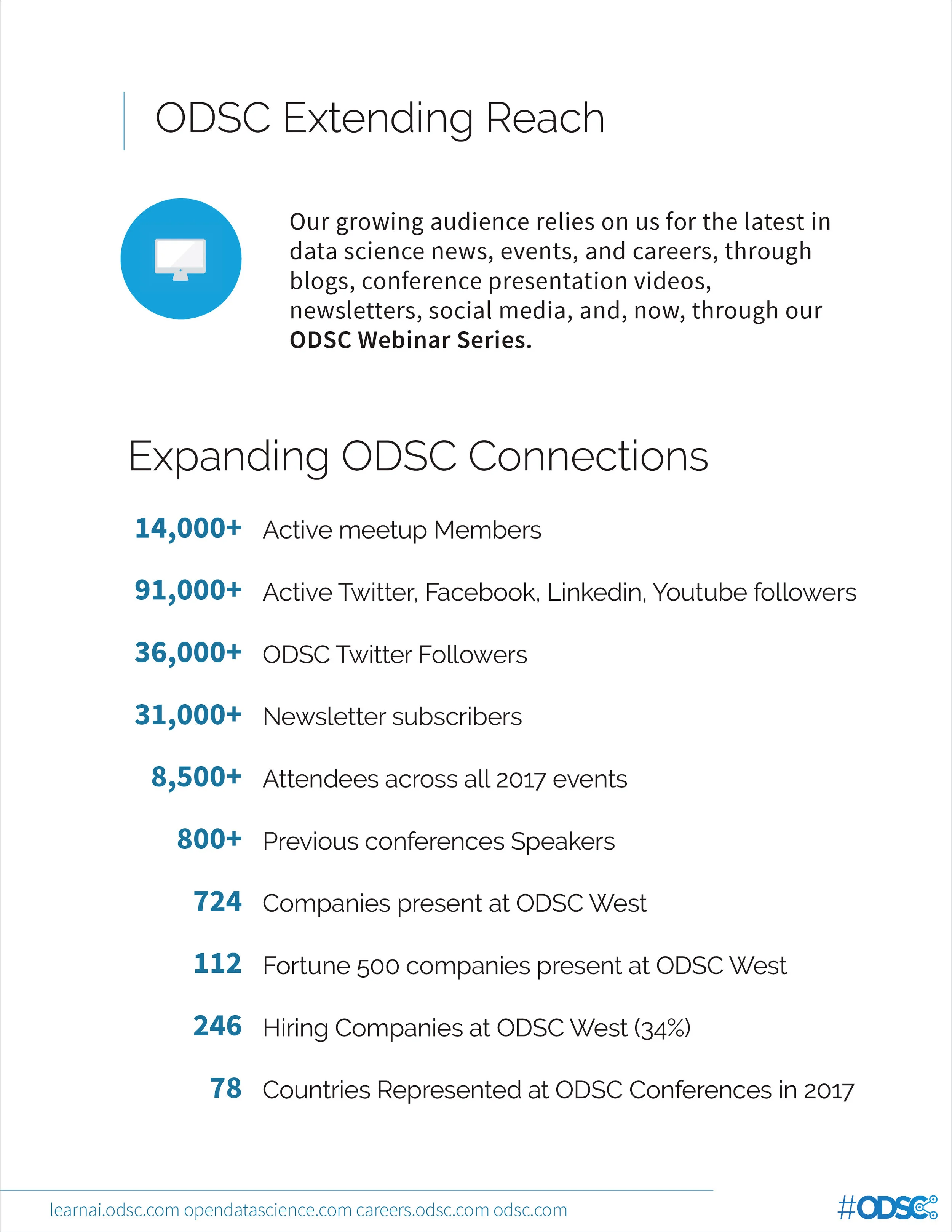
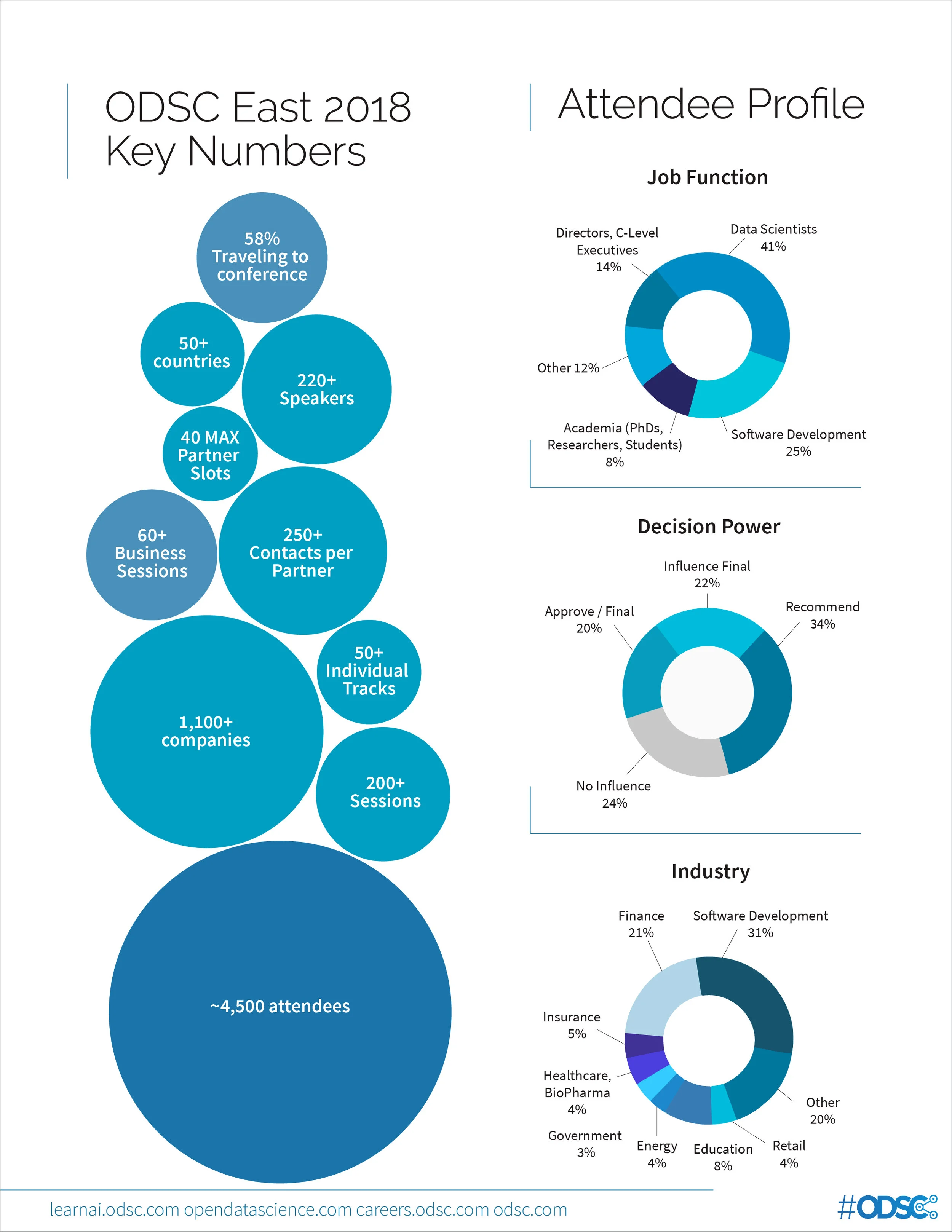


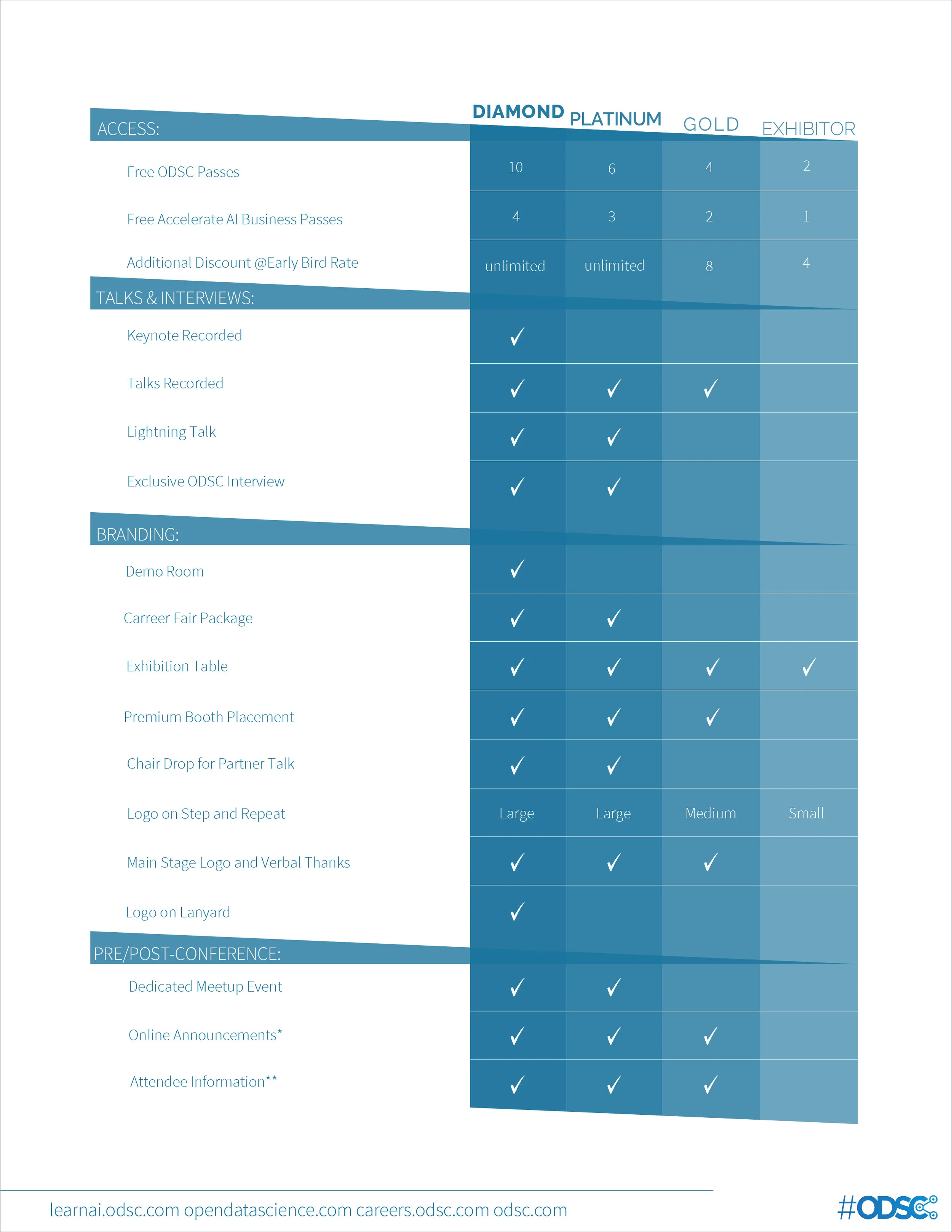
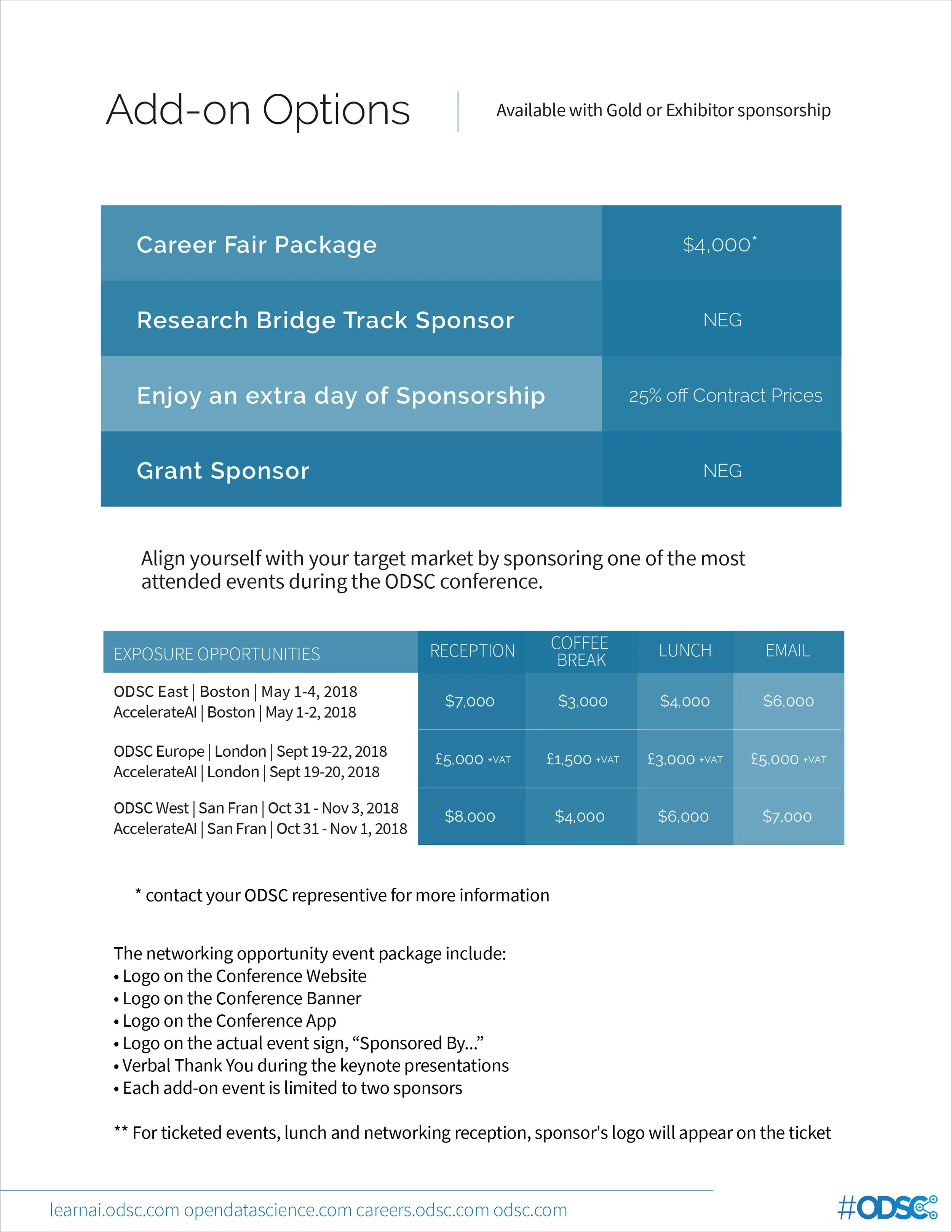

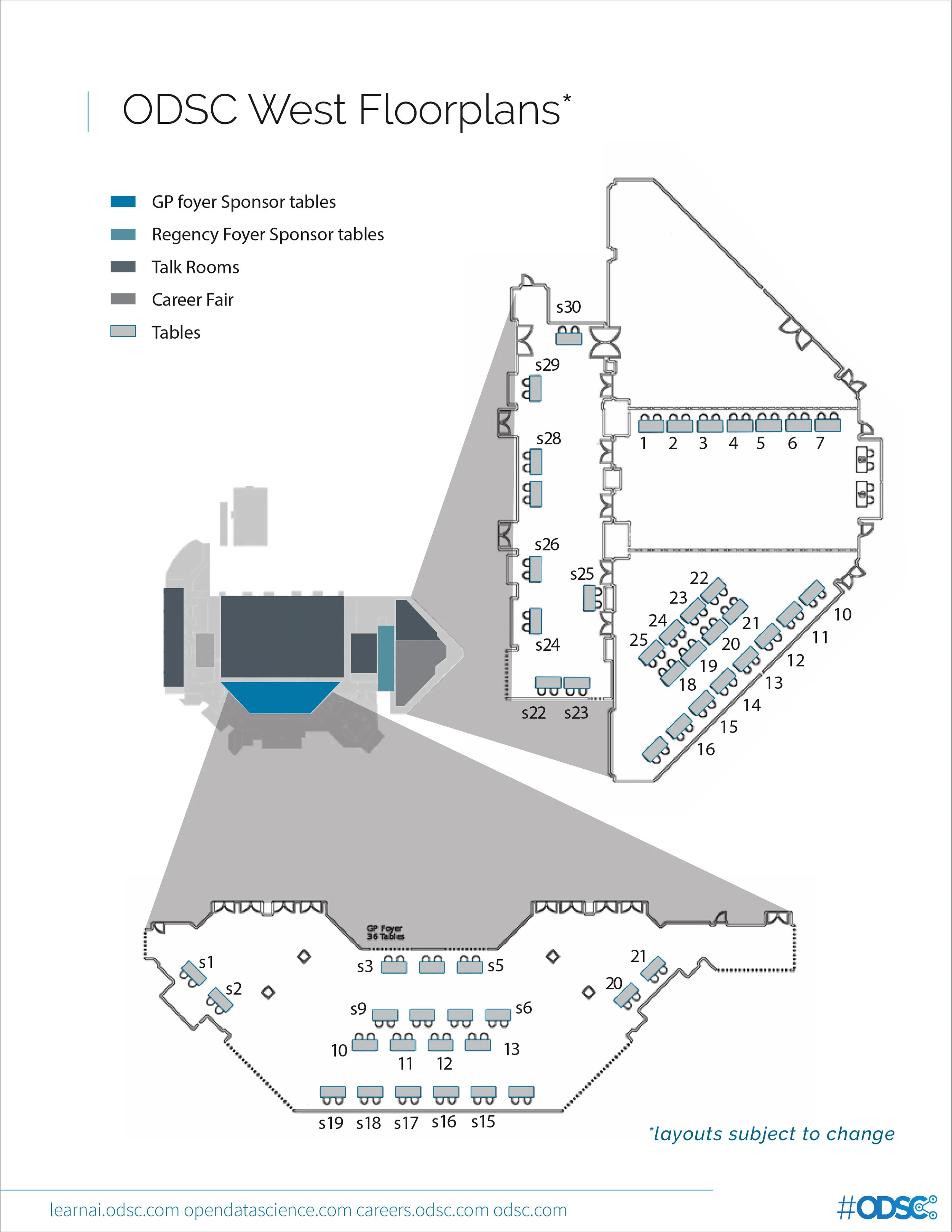
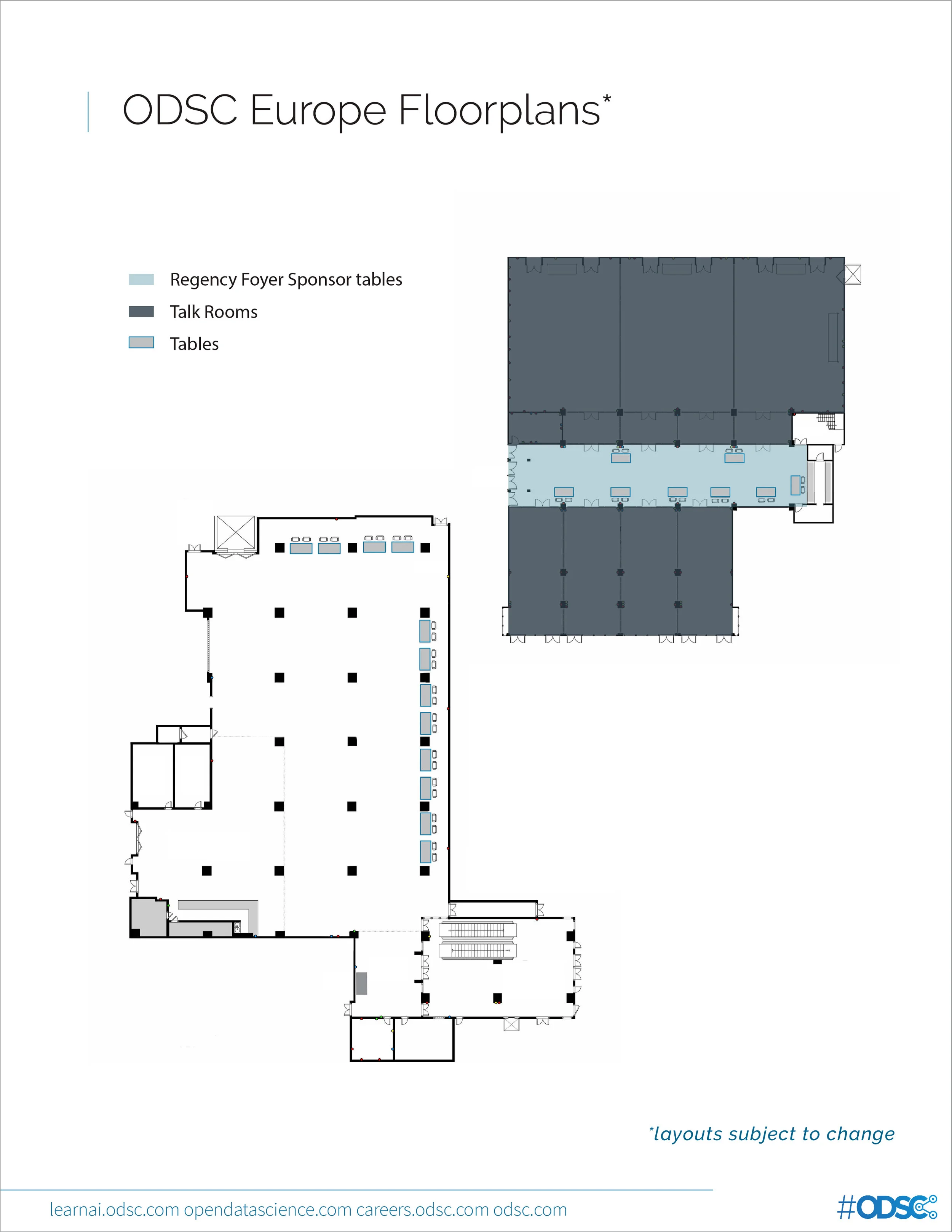
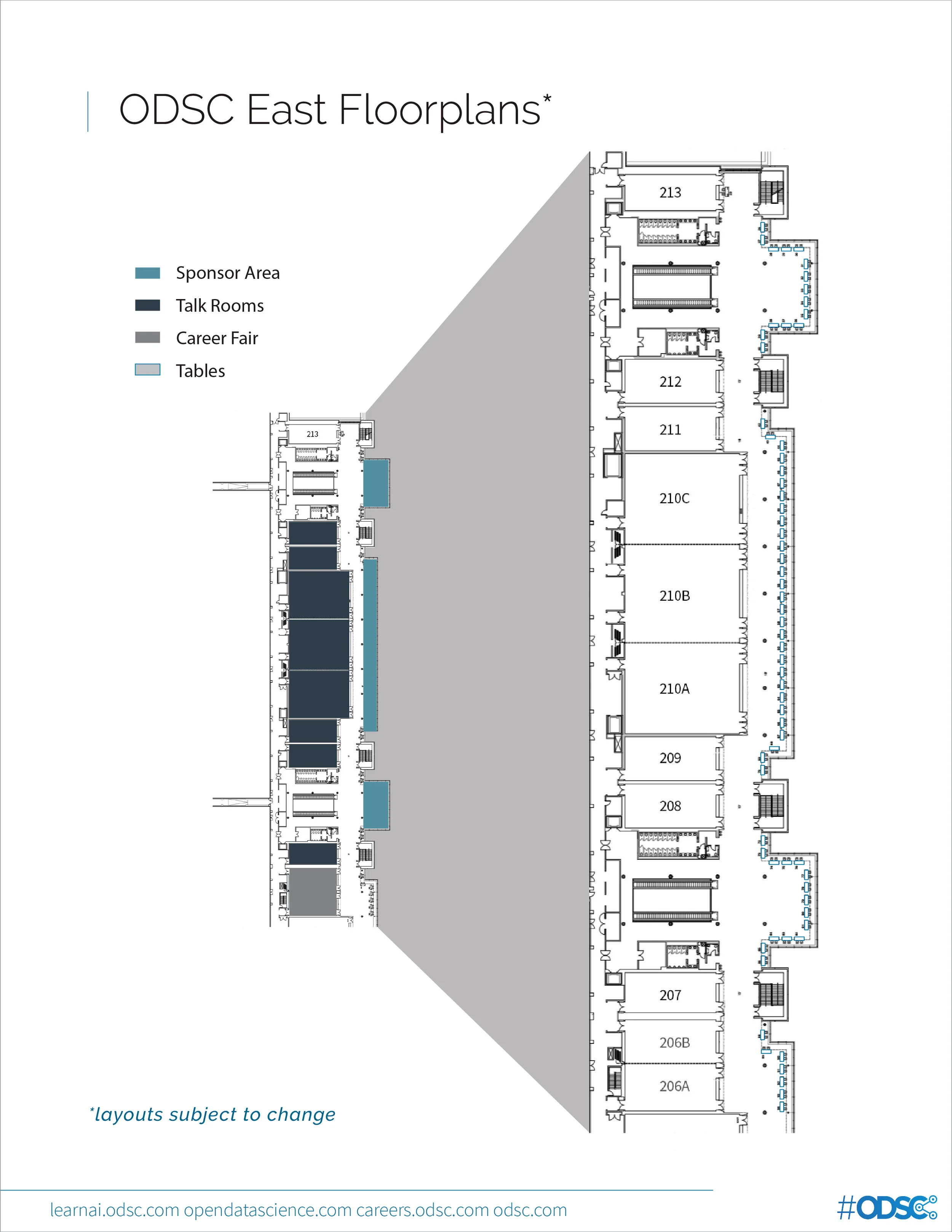
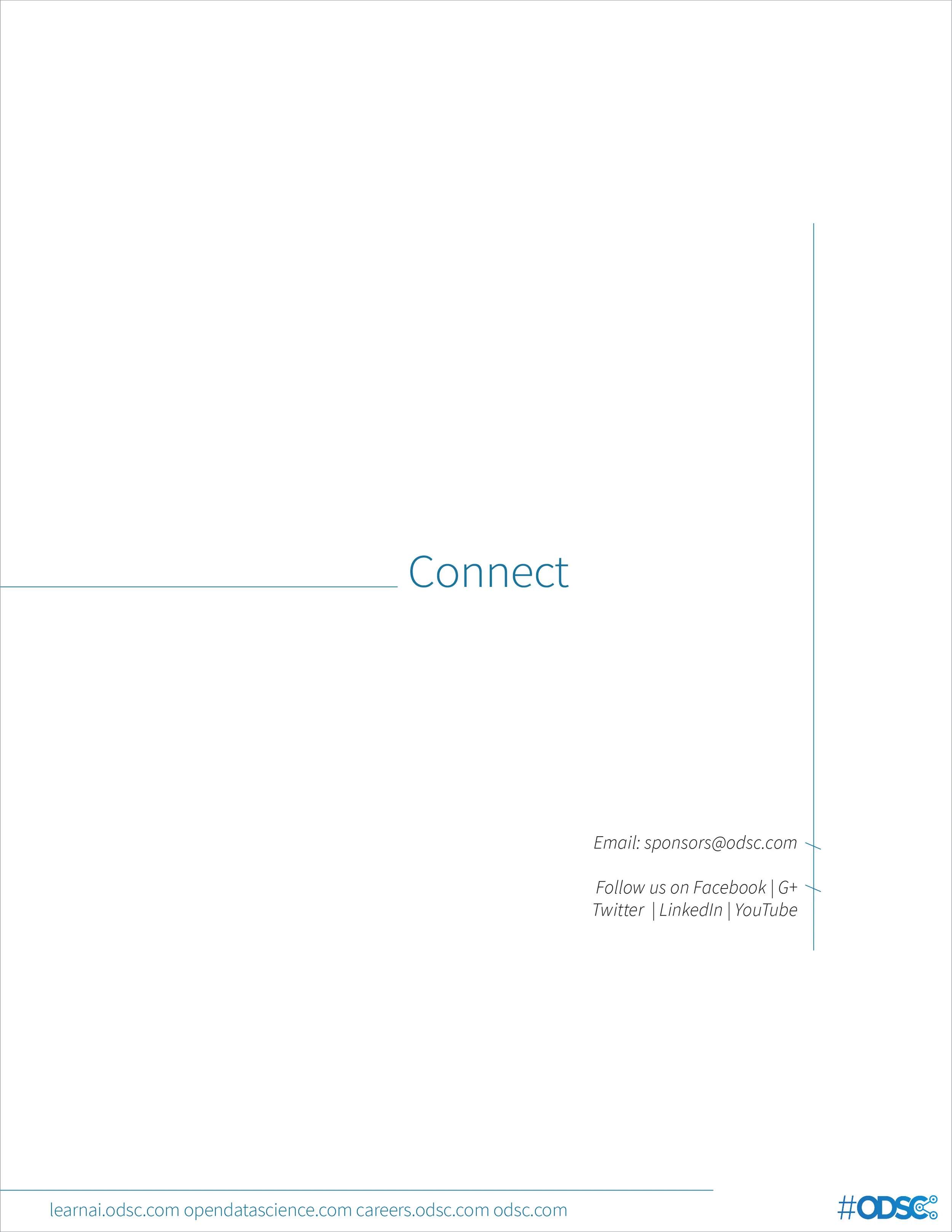
Content Design Example - interviewer, Diego Arenas
Content Example - written by Diego Arenas, original here.
ODSC State of Data Science 2017 report: The Data Wave

- Data Science roles
- Where the jobs are
- The going rate for Data Scientists
- The top 5 skills existing data scientists should learn and every new hire should have
view more
OVERVIEW
Data Scientists emerge from various backgrounds. Some hail from research fields like Biology, Chemistry and Physics while others rise from more quantitative backgrounds like Mathematics, Statistics and Economics, then there are those with Computer Science backgrounds, engineers… developers… journalists all stirring into the Data Scientist community. Some train themselves through experience, while others learn through academia. What they all have in common are two basic skills: a penchant for mathematics and above average analytical and critical thinking skills. During a great talk at ODSC Europe 2016 Neil Lawrence, Senior Principal Scientist at Amazon said, “There’s a sea of data, but most of it is undrinkable. So… who is a part of this desalination process?” This report expands on this metaphor in order to breakdown the field for those new to Data Science. Some may assumed that Data Scientists are to manage the entire wave of Big Data on their own, but this is not the case… growing are the careers, tools, and skills in the realm of data science. Companies do vaguely acknowledge this growth, and know that hiring in new capability will depend on their company’s needs, but understanding who to hire and why remains hugely preventative. Because some comp
THE DATA ENGINEER

THE BI DEVELOPER

THE DATA ANALYST

THE DATA SCIENTIST


see the rest
THE DATA SCIENCE TEAM

YOUR COMPANY

Companies interested in leveraging Data Science internally commonly start by redefining an existing data related role and start small. Employers define business goals for their data enabled employees, and grant them freedom to choose the analytical approaches to help them solve, like a programming language or a specific data analytics platform, but we’ll get into common tools and platforms later.
Assuming that companies using data science and their successes will incite others to ride the data wave, the job market will ache for data literacy. Just glancing at the number of data competitions, education programs and opportunities across industries, it seems it already is. Companies are literally stockpiling raw data in preparation for the oncoming storm, data is the new oil.
DATA SUPPORT SYSTEMS

ANALYSIS
Data Scientist Salaries in the US Cities
We crawled the website Indeed.com for job offers in the US cities and compared this year’s report to last years jupyter notebook written by Jason O’Rawe former ODSC Data Science Associate. It’s clear that most of the US jobs are still in the cities with heavy tech industries, so getting a Data Scientist seasoned or green to a job outside the hot spots might take a perky offer. See map belowThe average salary offered on Indeed.com for a “data scientist” job is 86.000USD. Average salary from state to state is consistent. For example, the average salary for a Data Scientist in the state of New York is only 4.000USD below the national average. The states with the most data scientist offers are California, New York, Massachusetts, Virginia and New Jersey.
The cities that have the most number of job postings, New York City and San Francisco, show average salaries over 125.000USD. Then, Seattle, Chicago, Boston and San José follow with an average salary over 115.000USD. The minimum average salary in any US city with more than 30 jobs posted on Indeed is ~80.000USD. It appears the US Data Science market is bull at least for aspiring data scientists.
The city with the highest average salary and with more than 30 job offers is New York City with an average of 126.000USD.
Data Science Skills
The most frequent data science skill in the posts analyzed is the fluency in the programming language Python. Its flexibility and multi-purpose scope helps data scientists clean data as well as building machine learning models and data visualizations. Tools like the Jupyter Project help to develop analysis in a lean and interactive way. The variety of libraries available is robust and the community behind Python makes it a baseline language.The R language is the second demanded skill required of data science positions in the US. Like Python, R also benefits from a community supporting its libraries.
R and Python may be the top two skills because users can test both locally on their personal machines, making them an accessible learning platform. Both languages have extensive libraries to support data analysis and modeling tasks. The development of frameworks with GUIs makes it even easier for new learners to jump in and swim with big players. We don’t see these two languages fading away in the near future, so put them on your job postings.
Number 3, ML, as a general term, is a key part in data science. Most of the data products will be a predictive model or the implementation of algorithms to understand the data, that’s why is mentioned in more than 50% of the job offers.
Fourth on the list, the SQL language is required to deal with queries to databases and form extractions or reports. SQL is an important part of extraction and modeling processes in a data science pipeline. If a data product is required as a final outcome, as some of the applications will be database oriented, SQL will be used to model and transmit the data to the persistent layer of a that data product.
Big Data skills are also required from data scientists, but expert proficiency in tools and frameworks seen throughout the chart like Hadoop and Spark, or Hive, Pig and HBase appear in a low percentage of posts meaning these competencies are required on a case by case basis. That being the current case, we expect to see growth for this spray of tools triggered by the increased access to IaaS (Infrastructure as a service), PaaS (Platform as a service) and cloud solutions making deployments feasible for companies with data science ambitions. New container technologies will yield new strategies on the use of big data platforms, and giving developers access will increase the user base and with it demand for these skills. The learning curve for data professionals is flattening with broad access to personal accounts in the cloud and to MOOCs. We are not sure the field will bow the way of eliminating coding as Patil and Davenport originally suggested, though drag and drop tools are trending fore filling their prediction, none the less Data Science is becoming more accessible, and on that point we are extremely proud.
Data Science Tools
If we parse the above skills by city and select for the top 5 cities according to number of job offers, we can see that positions in Boston and Chicago call for narrower skills sets; and in New York City, Seattle and San Francisco companies look for data scientists with a broader range of skills.On the graph below the tools are listed on the (x-axis) per city according to the key in the upper right. The number of times a skill appears in all the descriptions is noted as a percentage on the y-axis. Notice Chicago’s high percentage of job offers that include Python skills.
There is a trend toward a broad spectrum of data science tools over a dedicated focus. Compared with the previous year, the percentages are lower indicating that there may be a growing number of keywords in data science job specifications outside our search expectations.
As an aside, it is worth mentioning that Scala (number 7 on the list above) and Spark (number 8) skills were the top paid tech jobs in 2016, according to the stackoverflow’s Developers Survey 2016. Both programming language skills averaging $125,000.- In the same Developers Survey in 2017, Scala developers have the highest salaries with $110.000.- on average in the US.
Note: In later blogs and next years report we will broaden our scope to address the larger DS,